Learning Objectives
- Understand the importance of data management for successfully preserving data
- Review the Data Life Cycle and how it can guide the data management in a project
- Learn about metadata guidelines and best practices for reproducibility
- Become familiar with environmental data repositories for accessing and publishing data
- Introduce FAIR and CARE principles and the value it provides to data
- Evaluate the FAIRness and CAREness of your work and the work of others
The Big Idea
This lesson aims to get you thinking about how are you going to manage your data. And how to do it with the FAIR and CARE principles in mind. Even though you are in the early stages of defining you synthesis project, we believe that when it comes to data management, the earlier you start thinking about it the better. We also hope you can apply data management best practices and a FAIR and CARE lens to other research projects.
Introduction to Data Management
Data management is the process of handling, organizing, documenting, and preserving data used in a research project. This is particularly important in synthesis science given the nature of synthesis, which involves combining data and information from multiple sources to answer broader questions, generate knowledge and provide insights into a particular problem or phenomenon.
Benefits of managing your data
Successfully managing your data throughout a research project helps ensures its preservation for future use. It also facilitates collaboration within your team, and it helps advance your scientific outcomes.
From a researcher perspective
- Keep yourself organized – be able to find your files (data inputs, analytic scripts, outputs at various stages of the analytic process, etc.)
- Track your science processes for reproducibility – be able to match up your outputs with exact inputs and transformations that produced them
- Better control versions of data – easily identify versions that can be periodically purged
- Quality control your data more efficiently
- To avoid data loss (e.g. making backups)
- Format your data for re-use (by yourself or others)
- Be prepared to document your data for your own recollection, accountability, and re-use (by yourself or others)
- Gain credibility and recognition for your science efforts through data sharing!
Advancement of science
- Data is a valuable asset – it is expensive and time consuming to collect
- Maximize the effective use and value of data and information assets
- Continually improve the quality including: data accuracy, integrity, integration, timeliness of data capture and presentation, relevance, and usefulness
- Ensure appropriate use of data and information
- Facilitate data sharing
- Ensure sustainability and accessibility in long term for re-use in science
The Data Life Cycle
The Data Life Cycle gives you an overview of meaningful steps data goes through in a research project, from planning to archival. This step-by-step breakdown facilitates overseeing individual actions, operations and processes required at each stage. This is a visual tool that aims o help scientists plan and anticipate what will be the “data needs” for a specific project (Faundeen et al 2013) .
Primary Elements
Plan |
Map out the processes and resources for all activities related to the handling of the project’s data assets. Start with the project goals (desired outputs, outcomes, and impacts) and work backwards to build a data management plan, supporting data policies, and sustainability plans for each step. |
Data Management Plan (DPM) |
Acquire & Discover |
Activities needed to collect new or existing data. You can structure the process of collecting data upfront to better implement data management. Consider data policies and best practices that maintain the provenance and integrity of the data. |
Identifying data sources and mechanisms to access data |
Process |
Every step needed to prepare new or existing data to be able to use it as an input for synthesis. Consider the structure of the data, unit transformation, extrapolations, etc |
Cleaning & Wrangling data skills |
Integrate |
Data from multiple sources are combined into a form that can be readily analyzed. Successful data integration depends on documentation of the integration process, clearly citing and making accessible the data you are using, and employing good data management practices throughout the Data Life Cycle. |
Modeling & Interpretation |
Analyze |
Create analyses and visualizations to identify patterns, test hypotheses, and illustrate findings. During this process, record your methods, document data processing steps, and ensure your data are reproducible. Learn about these best practices and more. |
Modeling, Interpretation & Statistics |
Preserve |
Plan on how you are going to store your data for long-term use and accessibility so others can access, interpret, and use the data in the future. Decide what data to preserve, where to preserve it, and what documentation needs to accompany the data. |
Data packages & repositories |
Publish and Share |
Publication and distribution of your data through the web or in data catalogs, social media or other venues to increase the chances of your data being discovered. Data is a research product as publications are. |
DOIs and citations |
Cross-Cutting Elements
These elements are involved across all stages describes above. They need to constantly by addressed throughout all the Data Life Cycle, making sure effective data management is in place.
Describe |
Document data and each of the data stages by describing the why, who, what, when, where, and how of the data and processes. Metadata, or data about data, is key to data sharing and reuse. Documentation such as software code comments, data models, and workflows facilitate indexing, acquiring, understanding, and future uses of the data |
Metadata and documentation |
Manage Quality |
Employ quality assurance and quality control procedures that enhance the quality of data, making sure the measurements or outputs within expected values.Identify potential errors and techniques to address them. |
Quality Control and Quality Assurance techniques |
Backup and Secure |
Plan to preserve data in the short term to minimize potential losses (e.g., via software failure, human error, natural disaster). This avoids risk and ensures data is accessible to collaborators. This applies to raw and process data, original science plan, data management plan, data acquisition strategy, processing procedures, versioning, analysis methods, published products, and associated metadata |
Servers, secure data sharing services |
As you can see there is a lot happening around the Data Life Cycle. This is why PLANNING is a key first step. It is advisable to initiate your data management planning at the beginning of your research process before any data has been collected or discovered.
In order to better plan and keep track of all the moving pieces when working with data, a good place to start is creating a Data Management Plan. However, this is not only the starting point. This is a “living” document that should be consulted and updated throughout the project.
Data Preservation & Sharing
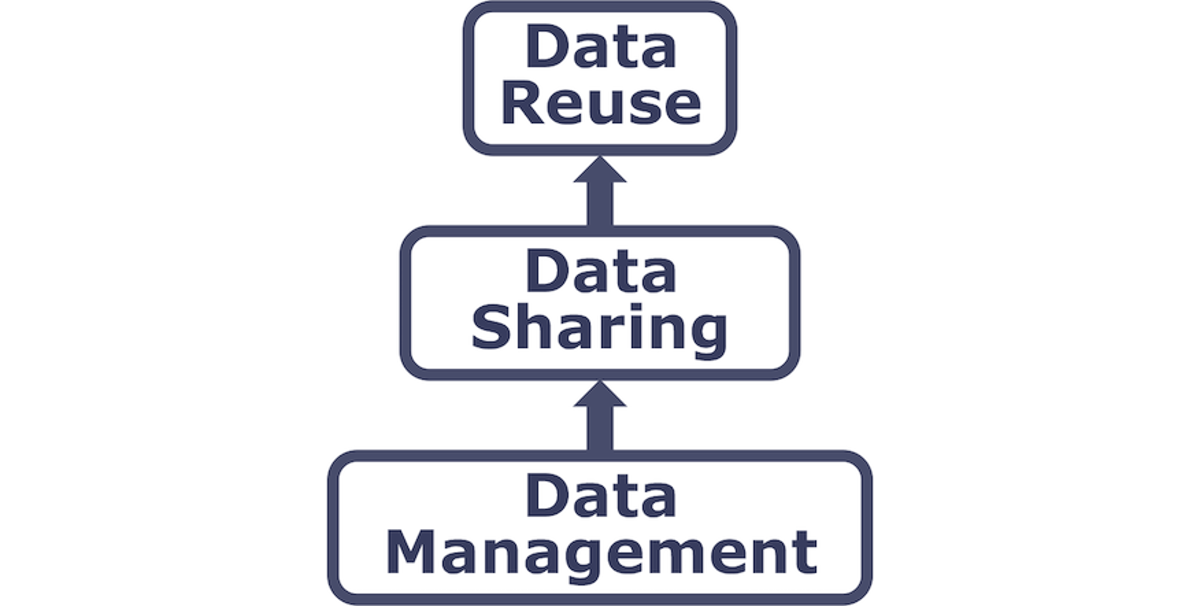
Data Packages
We define a data package as a scientifically useful collection of data and metadata that a researcher wants to preserve.
Sometimes a data package represents all of the data from a particular experiment, while at other times it might be all of the data from a grant, or on a topic, or associated with a paper. Whatever the extent, we define a data package as having one or more data files, software files, and other scientific products such as graphs and images, all tied together with a descriptive metadata document.
Many data repositories assign a unique identifier to every version of every data file, similarly to how it works with source code commits in GitHub. Those identifiers usually take one of two forms. A DOI identifier, often assigned to the metadata and becomes a publicly citable identifier for the package. Each of the other files gets a global identifier, often a UUID that is globally unique. This allows to identify a digital entity within a data package.
In the graphic to the side, the package can be cited with the DOI doi:10.5063/F1Z1899CZ
,and each of the individual files have their own identifiers as well.
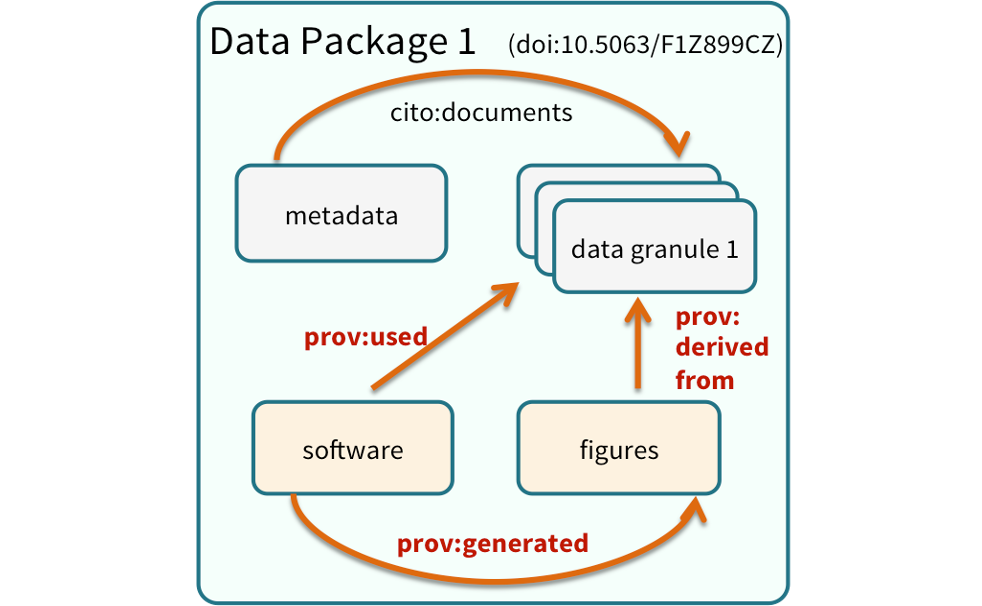
Data Repositories: Built for Data (and code)
- GitHub is not an archival location
- Examples of dedicated data repositories:
- KNB
- Arctic Data Center
- tDAR
- EDI
- Zenodo
- Dedicated data repositories are:
- Rich in metadata
- Archival in their mission
- Certified
- Data papers, e.g., Scientific Data
- re3data is a global registry of research data repositories
- Repository Finder is a pilot project and tool to help researchers find an appropriate repository for their work
DataOne Federation
DataONE is a federation of dozens of data repositories that work together to make their systems interoperable and to provide a single unified search system that spans the repositories. DataONE aims to make it simpler for researchers to publish data to one of its member repositories, and then to discover and download that data for reuse in synthetic analyses.
DataONE can be searched on the web, which effectively allows a single search to find data from the dozens of members of DataONE, rather than visiting each of the (currently 44!) repositories one at a time.
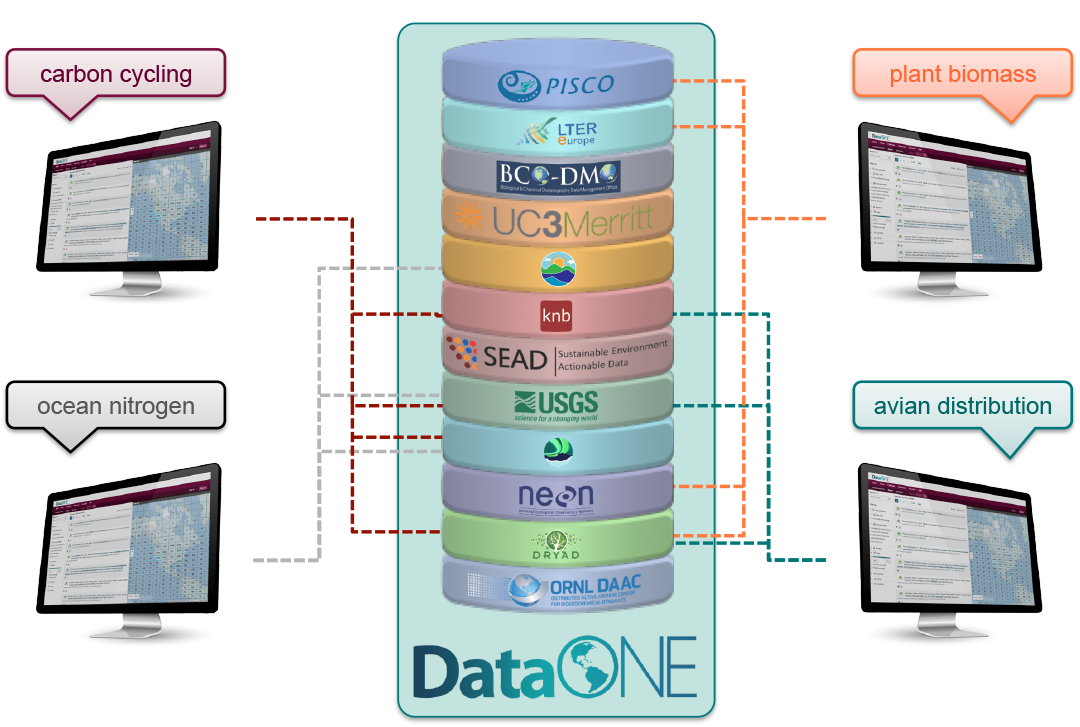
Data Management Summary
- The Data Life Cycle help us see the big picture of our data project.
- It is extremely helpful to develop a data management plan describing each step of the data life cycle to stay organized.
- Document everything. Having rich metadata is a key factor to enable data reuse. Describe your data and files and use an appropriate metadata standard.
- Identify software and tools that will help you and your team organize and document the project’s data life cycle.
- Publish your data in a stable long live repository and assign a unique identifier.
Now that we have talked about the big picture of data management, it is time to focus on the FAIR & CARE principles. It is important to learn about these principles and apply them since the very beginning of your planning process. This will make it easier to include and maintain tidy and ethical principles throughout the entire project.
The FAIR and CARE Principles
The idea behind these principles is to increase access and usage of complex and large datasets for innovation, discovery, and decision-making. This means making data available to machines, researchers, Indigenous communities, policy makers, and more.
With the need to improve the infrastructure supporting the reuse of data, a group of diverse stakeholders from academia, funding agencies, publishers and industry came together to jointly endorse measurable guidelines that enhance the reusability of data (Wilkinson et al. (2016)). These guidelines became what we now know as the FAIR Data Principles.
Following the discussion about FAIR and incorporating activities and feedback from the Indigenous Data Sovereignty network, the Global Indigenous Data Alliance developed the CARE principles (Carroll et al. (2021)). The CARE principles for Indigenous Data Governance complement the more data-centric approach of the FAIR principles, introducing social responsibility to open data management practices.
Together, these two principle encourage us to push open and other data movements to consider both people and purpose in their advocacy and pursuits. The goal is that researchers, stewards, and any users of data will be FAIR and CARE (Carroll et al. (2020)).
What is FAIR?
With the rise of open science and more accessible data, it is becoming increasingly important to address accessibility and openness in multiple ways. The FAIR principles focuses on how to prepare your data so that it can be reused by others (versus just open access of research outputs). In 2016, the data stewardship community published principles surrounding best practices for open data management, including FAIR. FAIR stands for Findable, Accessible, Interoperable, and Reproducible. It is best to think about FAIR as a set of comprehensive standards for you to use while curating your data. And each principle of FAIR can be translated into a set of actions you can take during the entire lifecycle of research data management.
(F) Findable |
Metadata and data should be easy to find for both humans and computers. |
(A) Accessible |
Once someone finds the required data, they need to know how the data can be accessed. |
(I) Interoperable |
The data needs to be easily integrated with other data for analysis, storage, and processing. |
(R) Reusable |
Data should be well-described so they can be reused and replicated in different settings. |
FAIR Principles in Practice
This is not an exhaustive list of actions for applying FAIR Principles to your research, but these are important big picture concepts you should always keep in mind. We’ll be going through the resources linked below so that you know how to use them in your own work.
- It’s all about the metadata. To make your data and research as findable and as accessible as possible, it’s crucial that you are providing rich metadata. This includes, using a field-specific metadata standard (i.e. EML or Ecological Metadata Language for earth and environmental sciences), adding a globally unique identifier (i.e. a Digital Object Identifier) to your datasets, and more. As discussed earlier, quality metadata goes a long way in making your data FAIR. One tool to help implement FAIR principles to non-FAIR data is the FAIRification process. This workflow was developed by GoFAIR, a self-governed initiative that aims to help implement the FAIR data principles.
- Assess the FAIRness of your research. The FAIR Principles are a lens to apply to your work. And it’s important to ask yourself questions about finding and accessing your data, about how machine-readable your datasets and metadata are, and how reusable it is throughout the entirety of your project. This means you should be re-evaluating the FAIRness of your work over and over again. One way to check the FAIRness of your work, is to use tools like FAIR-Aware and the FAIR Data Maturity Model. These tools are self-assessments and can be thought of as a checklists for FAIR and will provide guidance if you’re missing anything.
- Make FAIR decisions during the planning process. You can ensure FAIR Principles are going to implemented in your work by thinking about it and making FAIR decisions early on and throughout the data life cycle. As you document your data always keep in mind the FAIR lense.
What is CARE?
The CARE Principles for Indigenous Data Governance were developed by the International Indigenous Data Sovereignty Interest Group in consultation with Indigenous Peoples, scholars, non-profit organizations, and governments (Carroll et al. (2020)). They address concerns related to the people and purpose of data. It advocates for greater Indigenous control and oversight in order to share data on Indigenous Peoples’ terms. These principles are people and purpose-oriented, reflecting the crucial role data have in advancing Indigenous innovation and self-determination. CARE stands for Collective benefits, Authority control, Responsibility and Ethics. It details that the use of Indigenous data should result in tangible benefits for Indigenous collectives through inclusive development and innovation, improved governance and citizen engagement, and result in equitable outcomes.
(C) Collective Benefit |
Data ecosystems shall be designed and function in ways that enable Indigenous Peoples to derive benefit from the data. |
(A) Authority to Control |
Indigenous Peoples’ rights and interests in Indigenous data must be recognized and their authority to control such data be empowered. Indigenous data governance enables Indigenous Peoples and governing bodies to determine how Indigenous Peoples, as well as Indigenous lands, territories, resources, knowledge and geographical indicators, are represented and identified within data. |
(R) Responsibility |
Those working with Indigenous data have a responsibility to share how those data are used to support Indigenous Peoples’ self-determination and collective benefit. Accountability requires meaningful and openly available evidence of these efforts and the benefits accruing to Indigenous Peoples. |
(E) Ethics |
Indigenous Peoples’ rights and well being should be the primary concern at all stages of the data life cycle and across the data ecosystem. |
CARE Principles in Practice
Make your data access to Indigenous groups. Much of the CARE Principles are about sharing and making data accessible to Indigenous Peoples. To do so, consider publish your data on Indigenous founded data repositories such as:
Use Traditional Knowledge (TK) and Biocultural (BC) Labels How do we think of intellectual property for Traditional and Biocultural Knowledge? Knowledge that outdates any intellectual property system. In many cases institution, organizations, outsiders hold the copy rights of this knowledge and data that comes from their lands, territories, waters and traditions. Traditional Knowledge and Biocultural Labels are digital tags that establish Indigenous cultural authority and governance over Indigenous data and collections by adding provenance information and contextual metadata (including community names), protocols, and permissions for access, use, and circulation. This way mark cultural authority so is recorded in a way that recognizes the inherent sovereignty that Indigenous communities have over knowledge. Giving Indigenous groups more control over their cultural material and guide users what an appropriate behavior looks like. A global initiative that support Indigenous communities with tools that attribute their cultural heritage is Local Contexts.
Assess the CAREness of your research. Like FAIR, CARE Principles are a lens to apply to your work. With CARE, it’s important to center human well-being in addition to open science and data sharing. To do this, reflect on how you’re giving access to Indigenous groups, on who your data impacts and the relationships you have with them, and the ethical concerns in your work. The Arctic Data Center, a data repository for Arctic research, now requires an Ethical Research Practices Statement when submitting data to them. They also have multiple guidelines on how to write and what to include in an Ethical Research Practices Statement.
Exercise: Evaluate a Data Package on the EDI Repository
Explore data packages published on EDI assess the quality of their metadata. Imagine you’re a data curator!
You and your group will evaluate a data package for its: (1) metadata quality, (2) data documentation quality for reproducibility, and (3) FAIRness and CAREness.
- View our Data Package Assessment Rubric and make a copy of it to:
- Investigate the metadata in the provided data
- Does the metadata meet the standards we talked about? How so?
- If not, how would you improve the metadata based on the standards we talked about?
- Investigate the overall data documentation in the data package
- Is the documentation sufficient enough for reproducibility? Why or why not?
- If not, how would you improve the data documentation? What’s missing?
- Identify elements of FAIR and CARE
- Is it clear that the data package used a FAIR and CARE lens?
- If not, what documentation or considerations would you add?
- Elect someone to share back to the group the following:
- How easy or challenging was it to find the metadata and other data documentation you were evaluating? Why or why not?
- What documentation stood out to you? What did you like or not like about it?
- How well did these data packages uphold FAIR and CARE Principles?
- Do you feel like you understand the research project enough to use the data yourself (aka reproducibility?
If you and your group finish early, check out more datasets in the bonus question.
References
Carroll, Stephanie Russo, Ibrahim Garba, Oscar L. Figueroa-Rodríguez, Jarita Holbrook, Raymond Lovett, Simeon Materechera, Mark Parsons, et al. 2020.
“The CARE Principles for Indigenous Data Governance.” Data Science Journal 19 (1): 43.
https://doi.org/10.5334/dsj-2020-043.
Carroll, Stephanie Russo, Edit Herczog, Maui Hudson, Keith Russell, and Shelley Stall. 2021.
“Operationalizing the CARE and FAIR Principles for Indigenous Data Futures.” Scientific Data 8 (1): 108.
https://doi.org/10.1038/s41597-021-00892-0.
Wilkinson, Mark D., Michel Dumontier, IJsbrand Jan Aalbersberg, Gabrielle Appleton, Myles Axton, Arie Baak, Niklas Blomberg, et al. 2016.
“The FAIR Guiding Principles for Scientific Data Management and Stewardship.” Scientific Data 3 (1): 160018.
https://doi.org/10.1038/sdata.2016.18.